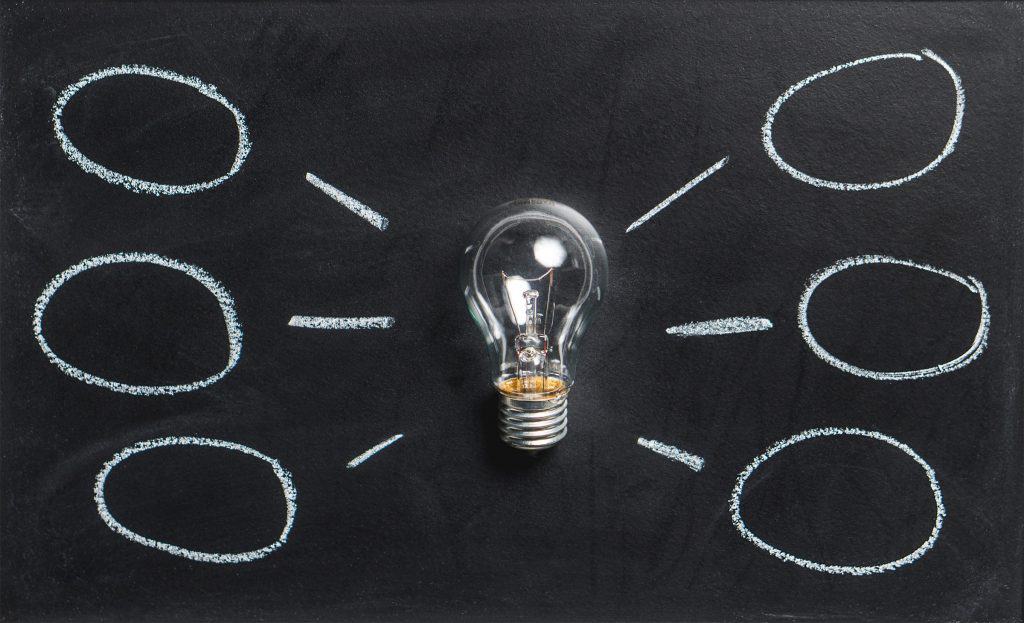
Detecting Sentiment and Emotion in Text
Recently I was thinking about how sentiment and emotion are reflected in written communication. Be that in copy for an e-commerce product page, a press release, a blog post, or the bio on your LinkedIn profile. Effective communication requires the receiver to accept your message with the same tone and sentiment you intended. Word choice and sentence structure can play a significant role in how your thoughts and received. Don’t get me wrong, there is a time and place for a negative tone in your writing but generally, you’re going to want to convey at least a neutral sentiment if not a positive one. For example, if you are looking for a job, you certainly don’t want someone to read your profile or resume and end up with a negative thought of you based on your communication style.
I explored the sentiment and emotion engines from Amazon Web Services, Microsoft Azure, Google Cloud Platform and IBM Cloud / Watson to see how they can be leveraged to improve online communication. You’ll read below in each service’s respective section about my experience with the platforms and the results of their natural language processing. I’ve also included links to appropriate resources, pricing info, and sample PHP code to interact with each API to get you started if you’d like to play with this yourself.
Overall, for content I wrote, I liked the feedback from IBM Watson the most, followed closely by AWS Comprehend. I found Azure’s lack of specificity and Google’s general approach to be lacking; too subjective. I understand all these engines will be somewhat subjective based on their training but when I see the results from IBM and AWS I feel a degree of confidence in the result based on the text I supplied. Just getting a number or 2 back from Azure and Google makes it feel like I must now do more work to interpret their result. In fact, Google says as much in their documentation. However, when I grabbed text off the Internet and plugged it into the APIs I was surprised to see the results. Shockingly different from what I expected in some cases.
Below are the 5 samples of text I used to text each of the platforms’ artificial intelligence services:
Sample #1 is from my LinkedIn bio:
I am a well-rounded, cloud focused, IT professional. I’m comfortable in any role from contributor to manager. I’m willing to grow within an organization to achieve the goal of returning to a manager/director role in a cloud-focused company.
My roles and interests encompass a wide range of responsibilities that provide me with expertise in many areas including cloud solutions offered by Amazon Web Services and Microsoft Azure, security, domains and DNS, web technologies (servers and development), and legal compliance. My experience also includes responsibilities for developing code to interact with cloud platform APIs, Iaas, DaaS, HPE Helion, CloudSystem 9 and 10, Scality, Stratoscale Symphony, Google Analytics, Google Adwords, etc.
Throughout my 10 years of leadership positions some of the numerous initiatives I have been responsible for include:
• Collecting and correlating data to design an anti-fraud solution that decreased fraud by 86%
• Reorganized and led a team of 45 agents increasing service levels from 45% to 90% in less than 6 weeks
• The design & implementation of a global load balancing solution that improved page load times by up to 500% depending on global locationProgram Management and technical project management has allowed me to work with world-class customers delivering complex multi-million-dollar solutions on a timely basis. In doing so, I work with numerous vendors and inside team members from executive management to professional services, engineers, and developers.
Sample #2 is a tweet from President Trump:
The Fake News Awards, those going to the most corrupt & biased of the Mainstream Media, will be presented to the losers on Wednesday, January 17th, rather than this coming Monday. The interest in, and importance of, these awards is far greater than anyone could have anticipated!
— Donald J. Trump (@realDonaldTrump) January 7, 2018
Sample #3 is another tweet from President Trump as I think you’ll be interested in seeing the different responses from the AI engines:
North Korean Leader Kim Jong Un just stated that the “Nuclear Button is on his desk at all times.” Will someone from his depleted and food starved regime please inform him that I too have a Nuclear Button, but it is a much bigger & more powerful one than his, and my Button works!
— Donald J. Trump (@realDonaldTrump) January 3, 2018
Sample #4 is the first post on this page:
I’ve always understood happiness to be appreciation. There is no greater happiness than appreciation for what one has- both physically and in the way of relationships and ideologies. The unhappy seek that which they do not have and can not fully appreciate the things around them. I don’t expect much from life. I don’t need a high paying job, a big house or fancy cars. I simply wish to be able to live my life appreciating everything around me.
Sample #5 is a longer post titled “Short Paragraph on Happiness”. I won’t list the text here to spare you some scrolling but you can find it here.
OK, let’s go on with the show and talk turkey about each of the respective services.
AWS Comprehend
Features
AWS offers the following features as part of its Comprehend natural language processing service. In addition to the overall sentiment detected, the Sentiment Analysis function will give you scores for each possible value to show you how certain it is of its decision, out to as many as 16 decimal places. I’ll be rounding that up a bit for the sake of your eyes. I only experimented with the Sentiment Analysis function.
Keyphrase Extraction: The Keyphrase Extraction API returns the key phrases or talking points and a confidence score to support that this is a key phrase.
Sentiment Analysis: The Sentiment Analysis API returns the overall sentiment of a text (Positive, Negative, Neutral, or Mixed).
Entity Recognition: The Entity Recognition API returns the named entities (“People,” “Places,” “Locations,” etc.) that are automatically categorized based on the provided text.
Language Detection: The Language Detection API automatically identifies text written in over 100 languages and returns the dominant language with a confidence score to support that a language is dominant.
Topic Modeling: Topic Modeling identifies relevant terms or topics from a collection of documents stored in Amazon S3. It will identify the most common topics in the collection and organize them in groups and then map which documents belong to which topic.
Pricing
Natural Language Processing requests are measured in units of 100 characters, with a 3 unit (300 characters) minimum charge per request. The free tier gives you access to 50,000 units of text (about 5 million characters) for each of the APIs per month with each additional unit starting at $0.0001 with significant cost reductions based on volume. Topic Modeling is the exception as it is priced per job.
I like Amazon’s price structure as it gives you quite a lot of wiggle room to play and remains inexpensive to continue using.
For complete pricing please following the appropriate link in the Resources box above.
Limits
For my tests, only the first limit really applied. It was sufficient for my needs and seems large enough to accommodate most tasks you’d need it for. I included all limits for reference:
- The maximum document size is 5,000 bytes of UTF-8 encoded characters.
- The maximum number of documents for the BatchDetectDominantLanguage, BatchDetectEntities, BatchDetectKeyPhrases, and BatchDetectSentiment operations is 25 documents per request.
- The BatchDetectDominantLanguage and DetectDominantLanguage operations have the following limitations:
- They don’t support phonetic language detection. For example, they will not detect “arigato” as Japanese, nor “nihao” as Chinese.
- They may have trouble distinguishing close language pairs, such as Indonesian and Malay; or Bosnian, Croatian, and Serbian.
- For best results, the input text should be at least 20 characters long.
API
With Amazon, you really have to install and use either the CLI or language-specific SDK. I used the PHP SDK and found it really easy to install and quick to code against – not to mention clean. For the authentication in the SDK to be successful please be sure your system’s clock/time is set correctly.
<?php // Setup and install your SDK first! For PHP you do that with composer require 'vendor/autoload.php'; // Provide you AWS API keys, the user for these keys need to be given permissions to Comprehend $aws_access_key = ''; $aws_secret_access_key = ''; // The text you want to analyze $text = ''; // Do the work and get your result $aws = new Aws\Sdk([ 'version' => 'latest', 'region' => 'us-east-1', 'credentials' => [ 'key' => $aws_access_key, 'secret' => $aws_secret_access_key, ], 'Comprehend' => [ 'region' => 'us-east-1' ], ]);; $comprehend = $aws->createComprehend(); $aws_result = $comprehend->detectSentiment([ 'LanguageCode' => 'en', 'Text' => $text ]); // $aws_result will be an array you can access for the returned data echo 'AWS Results' . PHP_EOL; echo 'Sentiment: ' . $aws_result['Sentiment'] . PHP_EOL; echo ' -Mixed: ' . $aws_result['SentimentScore']['Mixed'] . PHP_EOL; echo ' -Negative: ' . $aws_result['SentimentScore']['Negative'] . PHP_EOL; echo ' -Neutral: ' . $aws_result['SentimentScore']['Neutral'] . PHP_EOL; echo ' -Positive: ' . $aws_result['SentimentScore']['Positive'] . PHP_EOL; // Alternately, if you'd like to see the full set of data returned print_r($aws_result); ?>
Results
As mentioned above I’ve rounded the 14-16 decimal places up for readability. I am more than happy with the result of my LinkedIn bio. However, I’m amazed at the results of the 2 tweets. My personal interpretation of the sentiment or tone in both is considerably more negative or hostile. The 4th and 5th samples tend to make me believe that keyword overuse can manipulate the score.
Result | Sample #1 – LinkedIn | Sample #2 – Fake News Tweet | Sample #3 – Nuclear Tweet | Sample #4 – Happy Paragraph | Sample #5 – Happiness Article |
Overall Sentiment | NEUTRAL | POSITIVE | POSITIVE | POSITIVE | POSITIVE |
Mixed Score | 0.0109 | 0.0699 | 0.0586 | 0.1605 | 0.0328 |
Negative Score | 0.0209 | 0.2199 | 0.0458 | 0.1584 | 0.0038 |
Neutral Score | 0.8809 | 0.3176 | 0.4155 | 0.1159 | 0.0281 |
Positive Score | 0.0872 | 0.3926 | 0.4801 | 0.5652 | 0.9352 |
Azure Text Analytics
Resources: |
Text Analytics Product Page |
Text Analytics Documentation |
Text Analytics API Docs |
Text Analytics Pricing |
Features
Azure’s feature set in Text Analytics is considerably lighter than the competing services. It does what I was looking for so I can’t complain about that but if you’re looking for more functionality you may need to look at IBM or AWS.
Sentiment analysis
The API returns a numeric score between 0 and 1. Scores close to 1 indicate positive sentiment, and scores close to 0 indicate negative sentiment. Sentiment score is generated using classification techniques. The input features of the classifier include n-grams, features generated from part-of-speech tags, and word embeddings. It is supported in a variety of languages.
Key phrase extraction
The API returns a list of strings denoting the key talking points in the input text. We employ techniques from Microsoft Office’s sophisticated Natural Language Processing toolkit. English, German, Spanish, and Japanese text are supported.
Language detection
The API returns the detected language and a numeric score between 0 and 1. Scores close to 1 indicate 100% certainty that the identified language is true. A total of 120 languages are supported.
Pricing
The free tier gives you up to 5,000 transactions. Once you go beyond that mark be prepared to pay $75 for the next tier. There is no per transaction incremental charge so you’ll see big jumps in fees for potentially small changes in your usage. Each “document” analyzed for each API call will be considered a transaction.
Limits
I do like that Azure will let you push a lot of test through at one time to help reduce the likelihood of being rate limited. Their “document” size is in-line with Amazon’s limits as well.
- Maximum size of a single document 5,000 characters as measured by String.Length.
- Maximum size of entire request 1 MB
- Maximum number of documents in a request 1,000 documents
- The rate limit is 100 calls per minute. Note that you can submit a large quantity of documents in a single call (up to 1000 documents).
API
With a little Googling, I found a PHP SDK for Azure but it seems to only support the base IaaS services right now. I was going for consistency with using a single programming language in this post so I had to use Azure’s REST API. Authentication is handled via passing your API key via a header in your HTTP POST.
<?php // Provide your Azure API key to access the Text Analytics service $azure_key_1 = ''; $azure_endpoint = 'https://eastus.api.cognitive.microsoft.com/text/analytics/v2.0/sentiment'; // The text you want to analyze $text = ''; // Do the work and get your result $data = array( 'documents' => array( array( 'language' => 'en', 'id' => '1', 'text' => "$text" ) ) ); $data = json_encode($data); $azure = curl_init(); curl_setopt($azure, CURLOPT_URL, $azure_endpoint); curl_setopt($azure, CURLOPT_POST, 1); curl_setopt($azure, CURLOPT_RETURNTRANSFER, 1); curl_setopt($azure, CURLOPT_HTTPHEADER, array( "Ocp-Apim-Subscription-Key: $azure_key_1", 'Content-Type: application/json', 'Accept: application/json' )); curl_setopt($azure, CURLOPT_POSTFIELDS, $data); $response = curl_exec($azure); $azure_result = json_decode($response, true); curl_close($azure); // $azure_result will be an array you can access for the returned data echo 'Azure Results' . PHP_EOL; echo 'Sentiment Score: ' . $azure_result['documents'][0]['score'] . PHP_EOL; // Alternately, if you'd like to see the full set of data returned print_r($azure_result); ?>
Results
I’m not crazy about Azure’s over-simplified score. For me, it lacks a level of detail that I liked from both Amazon and IBM/Watson. The score is from 0 (negative) to 1 (positive) out to 14 decimal places that I’ve rounded up a bit for viewability. Again, I was satisfied with the score for my bio but the sample #3 score surprised me.
Result | Sample #1 – LinkedIn | Sample #2 – Fake News Tweet | Sample #3 – Nuclear Tweet | Sample #4 – Happy Paragraph | Sample #5 – Happiness Article |
Sentiment Score | 0.5 | 0.2960 | 0.5 | 0.1546 | 0.9891 |
Google Natural Language
Resources: |
Natural Language Product Page |
Natural Language Documentation |
Natural Language REST API Docs |
Natural Language Pricing |
Features
Google offers some interesting tools. Syntax Analysis and Entity Recognition look pretty cool although I’m not sure what practical use I would personally have for them. Alternately, I am surprised to see they don’t offer a keyword function.
Syntax Analysis
Extract tokens and sentences, identify parts of speech (PoS) and create dependency parse trees for each sentence.
Entity Recognition
Identify entities and label by types such as person, organization, location, events, products, and media.
Sentiment Analysis
Understand the overall sentiment expressed in a block of text.
Content Classification
Classify documents in predefined 700+ categories.
Multi-Language
Enables you to easily analyze text in multiple languages including English, Spanish, Japanese, Chinese (Simplified and Traditional), French, German, Italian, Korean and Portuguese.
Pricing
The Natural Language API is priced using units of measurement known as text records. A text record may contain up to 1,000 Unicode characters within the text content sent to the API for evaluation. Text in excess of these 1,000 characters counts as additional records. Prices are expressed in dollars per 1,000 text records (1,000,000 Unicode characters).
- Free up to 5K
- Over 5K “text records” processed cost depends on features used.
Limits
Google’s limits are based on text size, words/tokens in the text, and entity mentions. The API responds in different ways based on which limit you’ve exceeded. It’s not easy to describe so I’d recommend you read up on Googles Natural Language Quotas.
API
Google Cloud Platform does offer a PHP SDK but frankly, there were too many packages required to be installed to support it on my VM and I didn’t want to hassle with it. I decided to simply use their REST API instead. Your requests are authenticated by including your API key in the POST URI.
<?php // Provide your gcp API key to access the Text Analytics service $gcp_api_key = ''; $gcp_endpoint = 'https://language.googleapis.com/v1/documents:analyzeSentiment?key='; // The text you want to analyze $text = ''; // Do the work and get your result $data = array( 'document' => array( 'type' => 'PLAIN_TEXT', 'language' => 'en', 'content' => "$text" ), 'encodingType' => 'UTF8' ); $data = json_encode($data); $gcp = curl_init(); curl_setopt($gcp, CURLOPT_URL, "$gcp_endpoint$gcp_api_key"); curl_setopt($gcp, CURLOPT_POST, 1); curl_setopt($gcp, CURLOPT_RETURNTRANSFER, true); curl_setopt($gcp, CURLOPT_HTTPHEADER, array( 'Content-Type: application/json', 'Accept: application/json' )); curl_setopt($gcp, CURLOPT_POSTFIELDS, $data); $response = curl_exec($gcp); $gcp_result = json_decode($response, true); curl_close($gcp); // $gcp_result will be an array you can access for the returned data echo 'Google Cloud Platform' . PHP_EOL; echo 'Score: ' . $gcp_result['documentSentiment']['score'] . PHP_EOL; echo 'Magnitude: ' . $gcp_result['documentSentiment']['magnitude'] . PHP_EOL; // Alternately, if you'd like to see the full set of data returned print_r($gcp_result); ?>
Results
Google’s score is a value between -1.0 (Negative) and 1.0 (positive) and corresponds to the overall emotional leaning of the text. Strong positive and negative statements could balance each other out in the score. Generally, negative is -1.0 to -0.25, neutral is -0.25 to 0.25, and positive is 0.25 to 1.0.
Magnitude is from 0.0 to +inf. The higher the magnitude the stronger the positive or negative sentiment, based on the score. Lower magnitude indicates statements balancing others out, while higher values indicate the weight of the score overall.
While I suppose getting 2 scores to balance against is better than Azure’s response but because the Magnitude can extend to infinity it seems like there is far too much room to wonder about its value compared to scores for other blocks of text. However, I do like that Google breaks down your block of text into entities, or sentences, and gives you a score for each entity as well. I only show the overall document score below but you may want to check this service out if you want to see how each sentence fares and how it impacts your overall document score.
Result | Sample #1 – LinkedIn | Sample #2 – Fake News Tweet | Sample #3 – Nuclear Tweet | Sample #4 – Happy Paragraph | Sample #5 – Happiness Article |
Sentiment Score | 0.2 | 0 | 0 | 0.2 | 0.6 |
Magnitude | 6 | 1.7 | 0.2 | 3.2 | 20.2 |
IBM Cloud (Watson) Natural Language Understanding
Features
IBM’s feature set is the most mature among the services I tested. This shouldn’t be a surprise considering their success with Watson over the years and the training they’ve been able to apply to their artificial intelligence platform. This article only covers the sentiment and emotion functions.
Categories
Categorize your content using a five-level classification hierarchy. View the complete list of categories here.
Concepts
Identify high-level concepts that aren’t necessarily directly referenced in the text
Emotion
Analyze emotion conveyed by specific target phrases or by the document as a whole. You can also enable emotion analysis for entities and keywords that are automatically detected by the service.
Entities
Find people, places, events, and other types of entities mentioned in your content. View the complete list of entity types and subtypes here.
Keywords
Search your content for relevant keywords.
Metadata
For HTML and URL input, get the author of the webpage, the page title, and the publication date.
Relations
Recognize when two entities are related, and identify the type of relation.
Semantic Roles
Parse sentences into subject-action-object form, and identify entities and keywords that are subjects or objects of an action.
Sentiment
Analyze the sentiment toward specific target phrases and the sentiment of the document as a whole. You can also get sentiment information for detected entities and keywords by enabling the sentiment option for those features. As you’ll read below this maturity and ease of use applies to their API as well.
Pricing
Pricing depends on your overall IBM Cloud subscription plan. On the Lite (read free) plan you get up to 30,000 NLU items per month. Whereas if you upgrade to the paid Standard plan you are purely usage based on every item request, with discounts based on volume. I found it interesting that when you sign up for the Lite plan they don’t even ask for a credit card number.
An NLU item is based on the number of data units enriched and the number of enrichment features applied. A data unit is 10,000 characters or less. For example: extracting Entities and Sentiment from 15,000 characters of text is (2 Data Units * 2 Enrichment Features) = 4 NLU Items.
Limits
This was incredibly difficult to track down on the IBM website. Based on 30 July 2017 Release Notes, text greater than 50K chars will be truncated, the previous limit was 1 kilobytes (1024 bytes). Fifty thousand characters is considerably higher than the other services discussed in this post.
API
The IBM Cloud website only details their REST API so that’s what I used to code against. However, an after the fact search revealed there is a PHP SDK (https://github.com/CognitiveBuild/WatsonPHPSDK or https://github.com/ThomasIBM/php-sdk).
A nice plus for IBM’s API is that you can request multiple features in each API call. They still charge you per feature requested but it’s a lot more convenient to be able to ask once for everything you want rather than making individual calls for each function.
<?php // IBM will give you a specific username and password to access the NLU service $ibm_user = ''; $ibm_pass = ''; $ibm_endpoint = 'https://gateway.watsonplatform.net/natural-language-understanding/api/v1/analyze?version=2017-02-27'; // The text you want to analyze $text = ''; // Do the work and get your result $data = array( 'text' => "$text", 'features' => array( 'emotion' => array( 'document' => true ), 'sentiment' => array( 'document' => true ) ), ); $data = json_encode($data); $ibm = curl_init(); curl_setopt($ibm, CURLOPT_URL, $ibm_endpoint); curl_setopt($ibm, CURLOPT_POST, 1); curl_setopt($ibm, CURLOPT_RETURNTRANSFER, true); curl_setopt($ibm, CURLOPT_HTTPHEADER, array( 'Content-Type: application/json', 'Accept: application/json' )); curl_setopt($ibm, CURLOPT_USERPWD, "$ibm_user:$ibm_pass"); curl_setopt($ibm, CURLOPT_HTTPAUTH, CURLAUTH_BASIC); curl_setopt($ibm, CURLOPT_POSTFIELDS, $data); $response = curl_exec($ibm); $ibm_result = json_decode($response, true); curl_close($ibm); // $ibm_result will be an array you can access for the returned data echo 'IBM Cloud / Watson' . PHP_EOL; echo 'Sentiment: ' . $ibm_result['sentiment']['document']['label'] . ' ('. $ibm_result['sentiment']['document']['score'] . ')' . PHP_EOL; echo ' -Sadness: ' . $ibm_result['emotion']['document']['emotion']['sadness'] . PHP_EOL; echo ' -Joy: ' . $ibm_result['emotion']['document']['emotion']['joy'] . PHP_EOL; echo ' -Fear: ' . $ibm_result['emotion']['document']['emotion']['fear'] . PHP_EOL; echo ' -Disgust: ' . $ibm_result['emotion']['document']['emotion']['disgust'] . PHP_EOL; echo ' -Anger: ' . $ibm_result['emotion']['document']['emotion']['anger'] . PHP_EOL; // Alternately, if you'd like to see the full set of data returned print_r($ibm_result); ?>
Results
Sentiment score ranging from -1 (negative sentiment) to 1 (positive sentiment). Emotion scores ranging from 0 to 1 for sadness, joy, fear, disgust, and anger. A 0 means the text doesn’t convey the emotion, and a 1 means the text definitely carries the emotion.
Result | Sample #1 – LinkedIn | Sample #2 – Fake News Tweet | Sample #3 – Nuclear Tweet | Sample #4 – Happy Paragraph | Sample #5 – Happiness Article |
Sentiment Label | Positive | Negative | Positive | Positive | Positive |
Sentiment Score | 0.818693 | -0.209025 | 0.626316 | 0.186908 | 0.67631 |
Sadness Score | 0.09241 | 0.289947 | 0.266669 | 0.230824 | 0.085069 |
Joy Score | 0.531483 | 0.153109 | 0.030636 | 0.620183 | 0.776985 |
Fear Score | 0.029453 | 0.121715 | 0.408096 | 0.471154 | 0.032804 |
Disgust Score | 0.031956 | 0.494803 | 0.198308 | 0.11162 | 0.018848 |
Anger Score | 0.060409 | 0.197176 | 0.41175 | 0.084088 | 0.024855 |
I’d love to read your questions and comments on these services. How do you think you can use these in your business or project?